Research, reproducibility, and reliability. These are the keywords when it comes to good science. As scientists, we try to prove that our results are significantly different. My colleague wished there was a “Journal of Negative Results,” a journal in which tests that did not work were published. Therefore, people would not spend their time trying out experiments that other researchers had discovered to have negative results. Unfortunately, we often do not publish our results that do not have a “significant difference” and try to find a significant difference in our experiments that would make it worthy of publication.
This is where most researchers go wrong. They confuse basic statistical rules that make their results seem more significant and reproducible. Anyway, most of these methods are not statistically correct, so make sure you do not make the following statistical errors in your manuscript.
Common Statistical Errors
Control Group
When studying the effect of an intervention at various time points, you must have a control group. Many other variables may affect the experiments that you did not expect, for example, the subjects may have become accustomed to the research and reported the results more efficiently at the end than at the beginning of the project. Subjects also learn as they participate in the experiment and become more proficient over time. So this needs to be controlled. Control and intervention groups should have the same size and be randomly sampled at the same time.
Comparison of Two Effects Without Directly Comparing Them
This happens when the influence of two factors is compared with a control group but not with each other. For example, you have two groups and you want to compare two different interventions. Intervention A seems to be more effective than intervention B, but your statistical analysis proves that the two are not significantly different. However, you have found that each intervention is significantly different from the control group. The only conclusion you can draw is that both interventions show a significant difference, compared to the control group. You cannot say that the two interventions have a statistically significant difference.
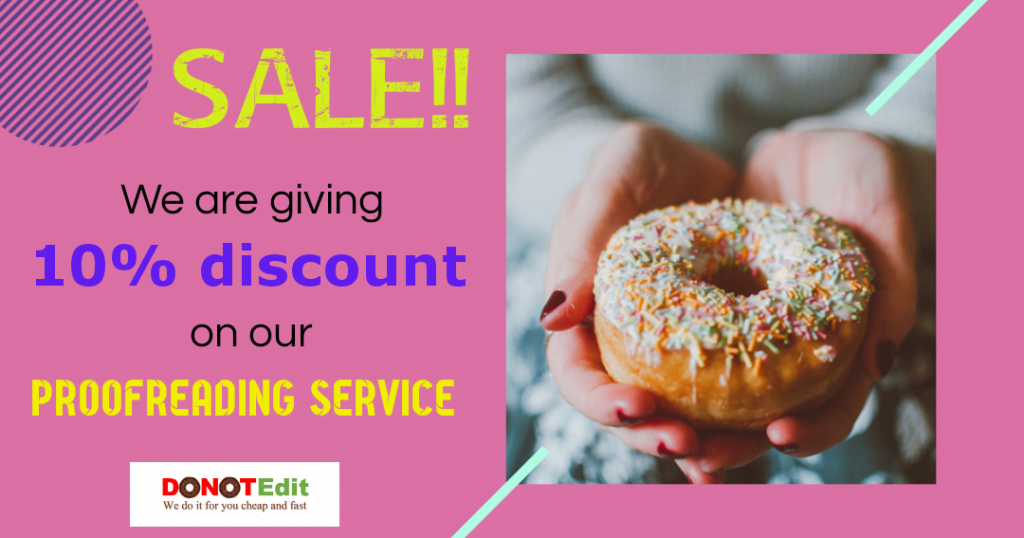
Number of Observations vs. Number of Subjects
The more you repeat your experiments, the more reliable your statistical analysis will be. Do not yield to the temptation to increase the number of units of analysis by using the number of observations instead of the number of subjects. To control yourself, look at the purpose of the experiments. If you want to examine the impact of an intervention on a group, then the unit of analysis should be the number of subjects.
Dealing with Outliers
You may think that you should skip a lot of outliers because something seems to be wrong with that data point. You might think that is it impossible for one or two of your data points to be so different from your other observations. Nevertheless, outliers can be reliable observations. If you delete a data point, you should mention and justify it. This kind of transparency is essential for good science.
Small Sample Sizes
In cases where the samples are rare and therefore limited, only large effects are statistically detectable. In addition, a credible impact may not be identified. If you find yourself in such a situation, you may be able to use the same small sample size by performing replications within and between samples and including a sufficient number of controls.
Imagine that you have completed a series of experiments by comparing the impact of an intervention before and after that intervention. You notice that the results between these two groups are not significantly different. However, during the analysis, you notice a change in the behavior of your samples and are tempted to divide your group into subgroups and set aside basic information to confirm your hypothesis. This is known as circular analysis or double-dipping and can be merely an observation of background noise. To confirm your hypothesis, you must repeat the experiment with new analytical criteria and appropriate control groups.
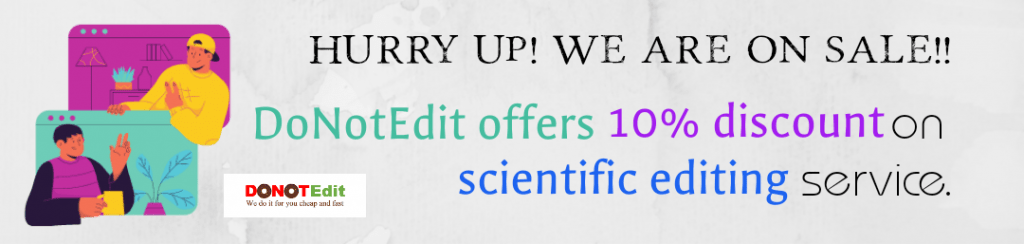
P-Hacking
This “flexibility of analysis” occurs when researchers artificially increase the probability of achieving a significant p-value by adding covariance, excluding subjects, or changing outcome parameters. This can give you a statistically significant result because the more tests you run, the more likely you are to get a false positive result. This is the way probability works. If you find yourself in such a situation, try to be transparent and use the available data to justify additional research.
No Correction for Multiple Comparisons
Multiple comparisons occur when two groups are compared using more than one variable. For example, you investigate the effect of a drug on volunteers and evaluate the outcome of several symptoms. In this type of test, the higher the number of factors, the greater the probability of a false positive for one of the variables. Make sure to correct multiple comparisons of a group with a larger set of variables using statistics from your experiment.
Over-Interpretation of Non-Significant Results
The significance of the p-value of 0.05 is optionally explained and only indicates the high probability of an outcome occurring in a population. For the p-value to be significant, it should be reported with the effect size and 95% confidence interval. In this way, the readers can better understand the scale of the effect and conclude whether they can predict the results for the relevant population based on the results of the sample population.
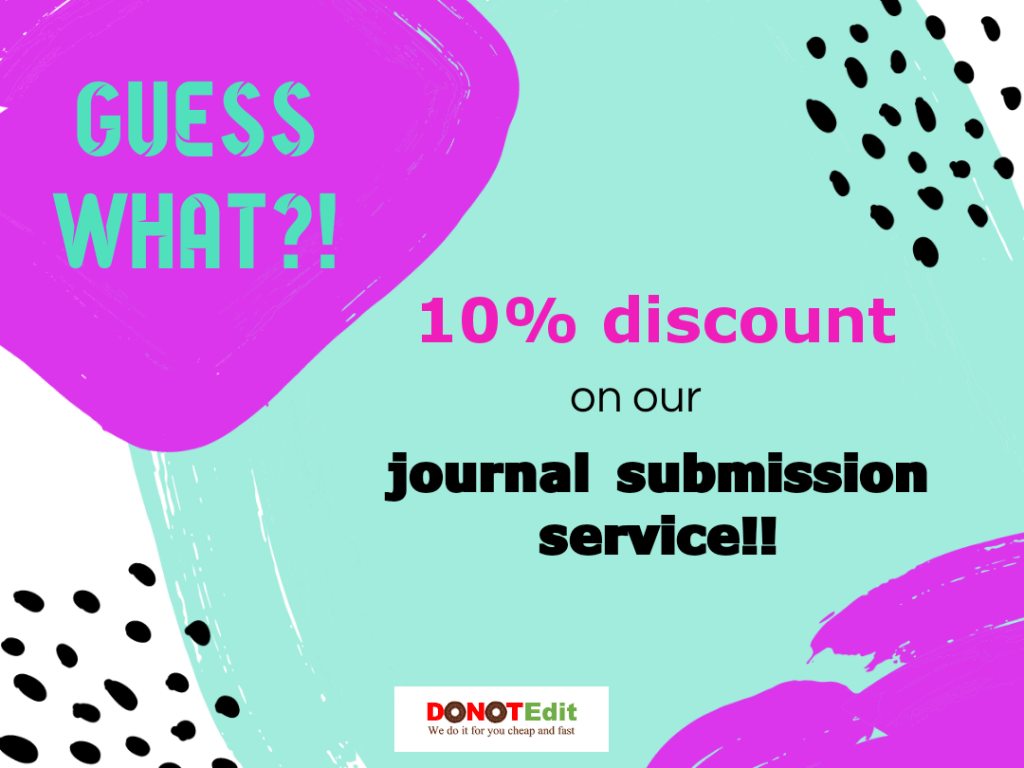
Confusing Correlation with Causal Relationship
The relationship between the two variables is often analyzed using correlations. If a correlation is found between two variables, it does not mean that one effect causes another. More experiments are needed to confirm the causal relationship.
Here are some tips that can help you:
- Plan for your research and goals before performing your tests.
- Define your population.
- Consider and all possible sources of variance and control for them.
- Choose statistical tests before you start your experiment.
- Include full details of your data and analysis in your report.
- Do not use the same data set to formulate hypotheses and test them.
- Be careful not to choose the wrong sample population and make sure to specify your population.
- Do not forget to select samples randomly and representatively.
- Do not use inappropriate statistical methods.
- Do not try to present your data by twisting your observations or trying a different statistical test that fits the hypothesis.
The purpose of statistical analysis is to tell the story of an intervention or influence that you are researching. You need to make sure you have all the parts of the story in order to draw significant conclusions. What statistical errors have you noticed while reading journal articles?