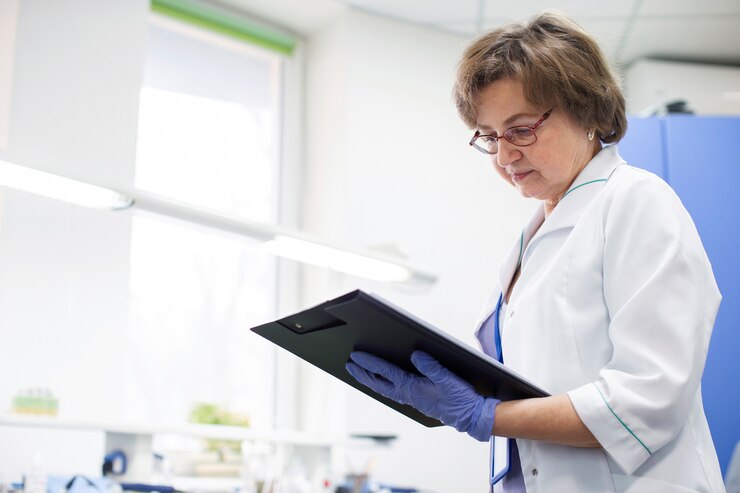
Scientists begin their research with the hypothesis that there is some kind of relationship between variables. The null hypothesis is the opposite of this hypothesis and states that there is no such relationship between the variables. The null hypothesis may not sound exciting, but it is a very important aspect of research that should not be overlooked. In this article, we discuss what the null hypothesis is, how it should be used, and why researchers should use it to improve their statistical analyses.
What is a Null Hypothesis?
A null hypothesis can be tested using statistical analysis and is often written as H0 (pronounced “H-naught”). Once you have determined how likely the sample relationship is if H0 is true, you can perform your analysis. Researchers use a significance test to determine the probability that the results indicating H0 are not due to chance.
Examples of Null Hypothesis and Alternative Hypothesis
The null hypothesis and the alternative hypothesis are not the same. The alternative hypothesis states that there is a relationship between two variables, while H0 states the opposite. You will understand this better by the following example.
A researcher wants to explore the relationship between the number of exercise sessions and appetite. He raises this question: Does increasing the number of exercise sessions lead to an increase in appetite?
Alternative hypothesis: increasing exercise sessions leads to increased appetite.
Null hypothesis: There is no relationship between the two variables. Increasing exercise sessions does not lead to increased appetite.
Consider another example of how to state a null hypothesis:
Question: Does insufficient sleep lead to an increased risk of a heart attack in men over 50?
Null hypothesis: lack of sleep in men over 50 does not increase the risk of a heart attack.
Why is Null Hypothesis Important?
Many scientists often neglect to use the null hypothesis in their research. As shown in the examples above, it is often assumed to be the opposite of the hypothesis being tested. However, it is good practice to apply this hypothesis in your research and ensure that it is carefully stated. To understand why, let’s go back to our previous example.
Alternative hypothesis: Lack of sleep leads to an increased risk of a heart attack in men over 50 years of age.
Null hypothesis: the amount of sleep in men over 50 years of age has no effect on the risk of a heart attack.
Note that this H0 is different from our first example.
Neither H0 nor the Alternative Hypothesis Is Supported
What should we do if we want to do this test and find that neither H0 nor the alternative hypothesis is supported?
The test is considered invalid. In this case consider our main H0, “The amount of sleep in men over 50 years of age does not increase the risk of a heart attack”. If this H0 is false and its alternative is also false, we can still consider the third hypothesis. Perhaps insufficient sleep actually reduces the risk of heart attack among men over 50. Because we have tested H0, we have more information than if we would have neglected it.
Do I Really Need to Test It?
The biggest problem with the null hypothesis is that many scientists consider its acceptance to be a failure of their experiment. They think they have not proven anything worthwhile. However, as we learned from the replication crisis, negative outcomes are just as important as positive outcomes. Although they may seem less attractive to publishers, they can provide important information to the scientific community about correlations that do or do not exist. In this way, they can advance science and avoid wasting resources.
Are you testing the null hypothesis? Why yes and why not? Share your thoughts with us in the comments below.